As many in the city planning twittersphere may know, the Bay Area Rapid Transit (BART) system’s twitter account has, from time to time, engaged customers in lively debate. In one particular instance earlier this year, BART responded to customer complaints over track maintenance that the system has had an unpredictable rise in ridership coupled with a lack of investment.
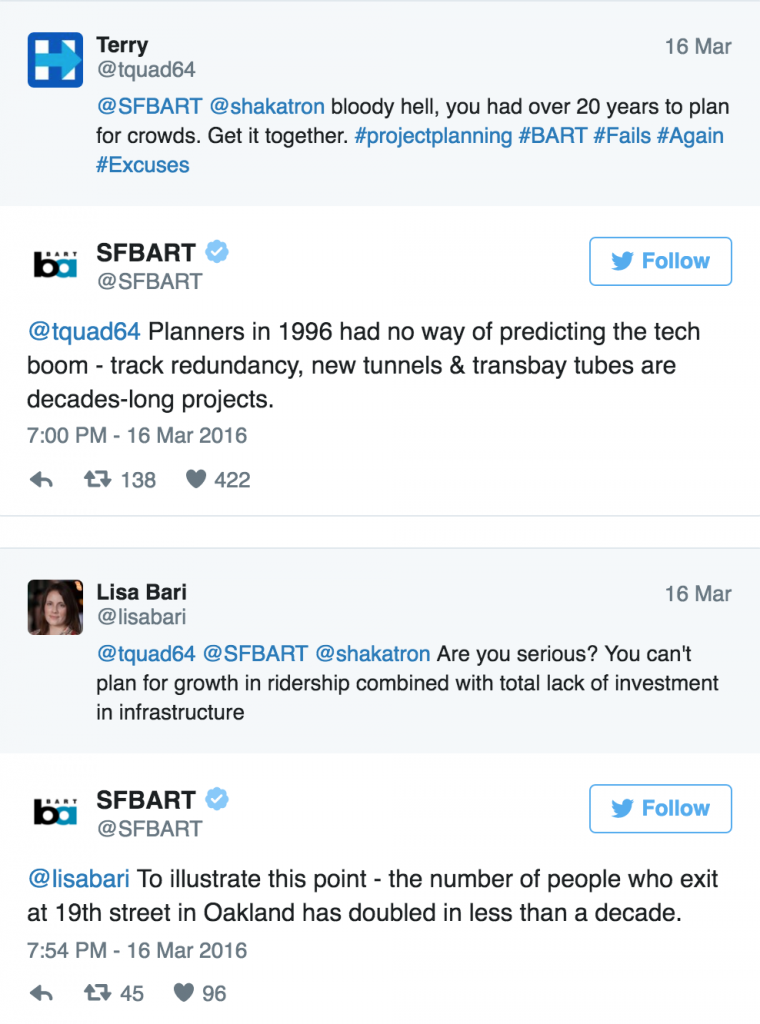
[ref]Screen shot taken from Upout.com (http://www.upout.com/blog/san-francisco-3/barts-twitter-account-getting-insanely-real-issues-lately)[/ref]
Leaving aside the state of BART’s infrastructure and investment, I want to take a closer look at BART’s ridership here. Exactly how much, and in which areas, has BART ridership increased? This simple exercise can help transportation planners determine both which stations serve the most people and where the ridership trends are heading. This, in turn, can serve as a metric to allocate limited resources. While this limited analysis does not fully answer these questions, it exposes some interesting trends to guide the inquiry, along with providing compelling evidence in favor of BART’s twitter defense.
First, let’s look at average weekday exits —defined as a single instance of a rider exiting a station—in 1999. I concentrate on weekday exits here because, anecdotally, workdays seem to generate the most customer frustration. While being late to a Sunday brunch is embarrassing, tardiness to a board meeting is probably worse. Furthermore, weekdays are when ridership is highest. As for 1999, this is the earliest year that I can find from BART’s weekday exit records. However, this works well because it roughly approximates the beginning of the tech boom mentioned in the twitter fight above.
Unsurprisingly, downtown San Francisco stands out as the single outlier on the map. While most stations in 1999 had around 5,000 exits per week, the Embarcadero and Montgomery stations downtown both had over 25,000. Also, the East Bay has fewer exits than the southern outskirts and of San Francisco—emphasizing that this area was the dominant commuting corridor at that time. The only exceptions to this are downtown Berkeley and the two downtown Oakland stations, all three of which had around 10,000 exits per week. This evidence certainly suggests that there was a relatively manageable set of heavily travelled stations. What does the map of 2016 exits look like, you ask?
Ridership grew almost everywhere throughout the system. Traditional heavyweights such as downtown San Francisco, Oakland, and Berkeley grew even bigger, with the top two stations—Embarcadero and Montgomery stations—now accounting for over 45,000 exits per weekday. Perhaps most importantly to the service quality of BART, the outskirts of major cities and suburbs grew sizably (with several new stations appearing). Not only could this put more strain on an aging system, but it also spreads track use over a wider area, making quality of service more and more difficult to maintain.
Finally, what do the numbers say in percentage terms?
Viewed through this lens, downtown San Francisco is far from king of the system. While there is still a wide gap in terms of absolute number of exits, Oakland, Berkeley, and the East Bay suburbs all equal or exceed SF in growth of ridership. Also, the outlying areas of the system are booming! More and more riders are taking longer rides, which surely puts strain on the system. Whoever is in charge of the BART twitter account was not lying. Riders may want to take it easier on a system that, built 60 years ago, has experienced dramatic growth in ridership over such a short period.
Data Sources and Decisions
Average weekday exit data by station comes from BART’s Ridership Reports page, found here. Additionally, geospatial data (kml file format) for the BART system comes from the BART website here. Unfortunately, matching on a string name variable was necessary to merge the geospatial data with the weekday exit data. There is a python script that includes a dictionary for this matching process at my github page here. In sum, I export a csv file with a crosswalk between the two datasets and then merge them within Carto. Also in the python script, I generate the percent change variable used in the above figures.
I made a number of data decisions within Carto during the mapping process. First, I chose a dark basemap and bright colors for the stations for contrast. I also made sure that the basemap’s labels do not overshadow the station points layer. In order to visualize the data, I chose a bubble map to compare an attribute—namely, station exits—of a points layer.
The BART station has expanded over the period 1999-2016, and the BART maps reflect this. I have purposely excluded stations that did not exist in 1999 from the 2016 map. One station, Warm Springs, is due to open in the Fall of 2016 and has been excluded from all maps. Although track expansions after 1999 are present on the 1999 map, I have decided to include the full extent of the present-day BART track system for two reasons: 1) the tracks layer is not split up into expansions, which makes it impossible to drop the expansion portions without dropping the whole system 2) I felt that the tracks were illustrative of the expanse and direction of the present-day BART system, reinforcing my overall point about BART’s enormous growth.
As for the bubble maps, I decided to use equal interval sizes for the bubbles. This is mainly because it easier for the reader to understand. Furthermore, the data in percentage terms is fairly uniformly distributed while the exit maps in absolute numbers have large outliers, which would be lumped together with much smaller stations in some other distribution.
Finally, I decided to label stations because I mention some specific stations in the article. Also, some of my readers might be unfamiliar with the BART system. However, labels would clutter the map at first glance, so I have them only appear at a specified zoom level.